New Ideas and Algorithms for Real-time, Real-world Machine Learning
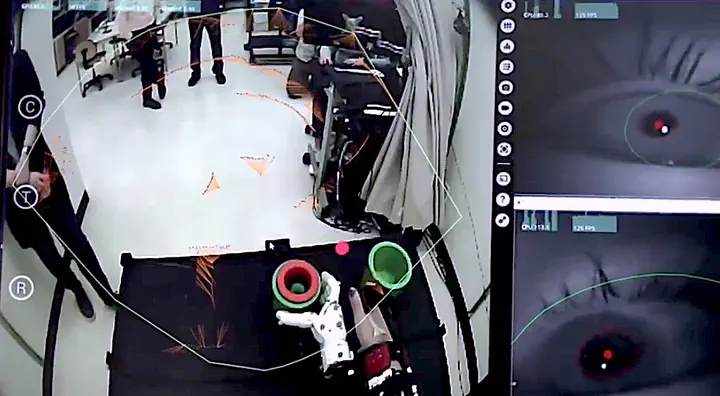
Our work on continual and contextual coadaptation of a prosthesis to its user has been largely rooted in the machine learning of forecasts of future sensorimotor outcomes through processes of temporal-difference learning (see Pilarski et al. 2017). A main fundamental investigation has therefore been extending and enhancing the idea of Generalized Value Functions (GVFs) and the Horde architecture initially proposed in Sutton et al. (2011). One major contribution has been new algorithms for GVF stepsize adaptation and feature selection, namely the extension of supervised learning stepsize adaptation methods to the RL setting in the form of Temporal-Difference Incremental Delta-Bar-Delta (TIDBD) (Kearney et al. 2019), TIDBD’s use in robot domains for tuning-free knowledge architectures and detecting broken or stuck robotic actuators (Gunther et al. 2020), and finally, an off-policy variant of TIDBD and its use in evaluating knowledge acquired by a learning agent (Kearney et al. 2022).
We have further uncovered approaches that allow GVF architectures to estimate their own introspective measures of surprise and form predictions of surprise (Gunther et al. 2018), and introduced the use of metagradient descent to select GVFs used in predictive-representation-of-state-based policy learning (Kearney et al. 2022). We have also pioneered new methods that allow GVFs to be learned faster and with more flexibility from real-world data streams, including Gamma-Nets (Sherstan et al. 2019) and accelerating GVF learning in constructive predictive frameworks via the successor representation (Sherstan et al. 2018).