AI Applied to Transforming the Science and Art of Prosthetic Restoration
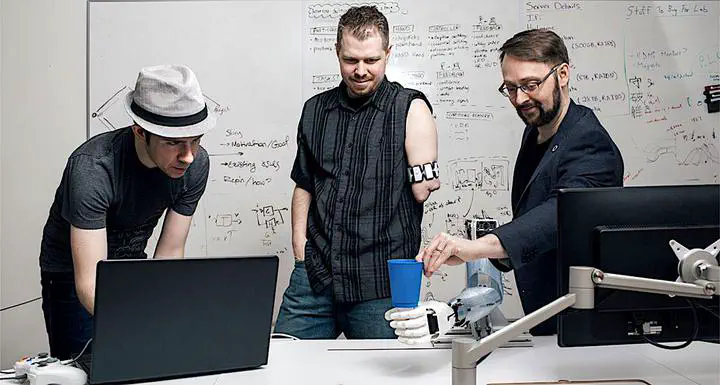
Our BLINC Lab is an interdisciplinary initiative focused on creating intelligent artificial limbs to restore and extend abilities for people with amputations. As part of this research, our team has developed and made prominent machine learning techniques for continual sensorimotor control and prediction learning on prosthetic devices. These include some of the first published approaches to ongoing user training of upper-limb prosthesis control systems via reinforcement learning, and we have pioneered the use of generalized value functions (GVFs) in prediction learning to continually adapt prosthetic control interfaces in real time. Of note are our Adaptive and Autonomous Switching algorithms (Edwards et al., 2016, and as contextualized in Pilarski et al. 2017 and Shehata et al. 2022): methods that adapt switching-based control interfaces based on learned, temporally extended predictions of what prosthetic function a user will select and when they will select it. These were arguably the first continual machine learning methods to be studied during use by someone with an amputation.
We followed this work with extensive studies of participants with and without amputations teaching a prosthetic limb how to coordinate its joints through reinforcement learning from demonstration (Vasan et al. 2017 & 2018), seminal results on continual machine learned feedback from a limb to a user (Parker et al. 2019), and automatic joint synergies (Brenneis et al. 2018 & 2019). We have conducted focused work on representations to support temporal-difference learning by a robotic prosthesis, creating a method known as Selective Kanerva Coding (Travnik et al. 2017) that proved highly suitable for use with brain-body-machine interfaces with constrained computational capabilities; this method has since been used by other groups for spinal microstimulation to enable standing and walking in live animals, and has been coupled with our adaptive switching methods for voluntary human exoskeleton control (Faridi et al. 2022). We have also pushed forward a next generation of nonlinear representations to help solve known contextual discrimination problems in prosthetic control, specifically our work on recurrent convolutional deep neural networks for solving the limb position effect (Williams et al. 2022a&b).
Our collaborative team has also produced the new gold-standard for evaluating human use of prosthetic technologies: the Gaze and Movement Analysis (GaMA) protocol (Hebert et al. 2019; Williams et al. 2019) and related software for assessing the functionality, cognitive demand, and biomechanical impact of novel intelligent assistive technologies like upper-limb robots and future osseointegrated (bone implanted) robots. This was the topic of extensive publications by our team (e.g., Valevicius et al. 2018, 2019, 2020, Lavoie et al. 2018, Boser et al. 2018, Williams et al. 2019, Hebert et al. 2019). GaMA has been used for regulatory approvals, and seen active uptake in industry, with research organizations, in clinics, and was the foundation for a spinoff company (GaMA Inc). GaMA now powers our own ongoing research to rigorously study machine learning in prostheses, eg. Brenneis et al. (2019) and Williams et al. (2022).